Building Enterprise Trust in a World of Generative AI
A forewarning to business leaders who adopt AI too quickly without understanding how to minimize risk
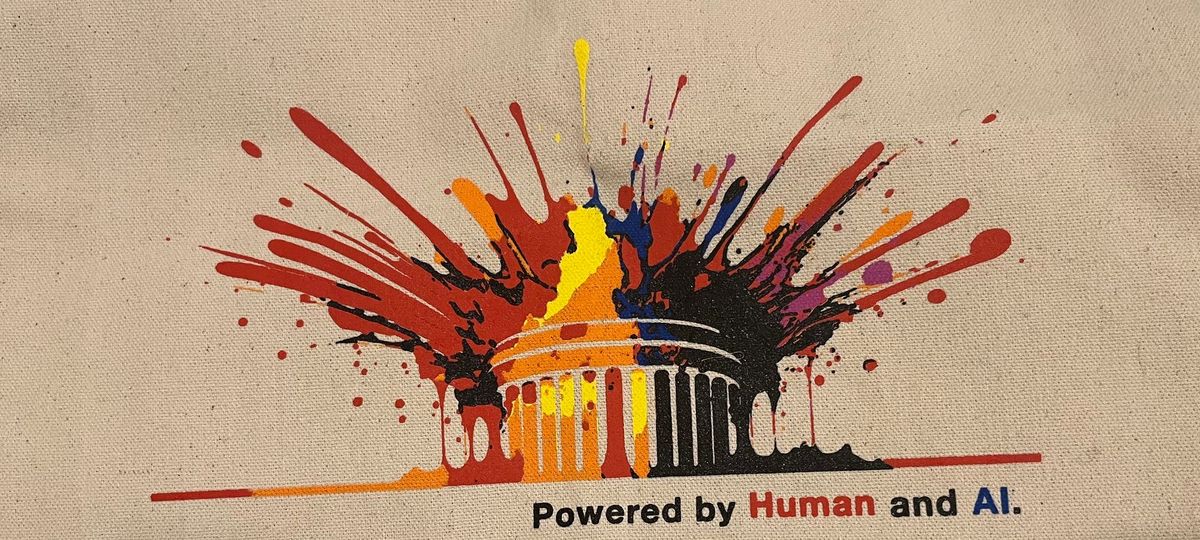
Last week was the inaugural MIT Generative AI Summit and it proved the following: AI is ready to be deployed at the forefront of enterprise applications and digital experiences. However, AI’s readiness does not allow for ignoring the associated risks. Organizations that will thrive in the intelligence generation will have to understand AI not just in the engineering context, but also in how it will affect their customers, employees, and their respective regulatory agencies.
This blog post is intended for business and engineering leaders considering how Generative AI will help a company’s frontline without compromising customer success. We’ll explore:
- Why businesses need to build trust with their customers and agents in order to augment their customer success departments with AI assistants
- The risks associated with Generative AI creating inaccurate or deceiving content
- The impacts AI will have outside of engineering for legal, risk, and diversity departments
Trust
“Trust” in the enterprise market boils down to the confidence your customers, employees, and shareholders have that you will respect and protect sensitive data and interactions. Technology continues to mediate more interactions that customers have with a business. As the tools have progressed, it’s becoming increasingly difficult to differentiate between a human and an AI speaking.
But does that mean we automatically have to engage with Hal? We need to create better definitions of when an AI should complete an entire interaction and when we need a human in the loop. With Generative AI, we now have the capability to create hyper-personalized responses with the customer’s previous interactions as fodder. Just to demonstrate the talent of Generative AI with minimal input, I’ve added an example from ChatGPT below:
This content is nearly indistinguishable from what a human agent would write back to the consumer. And when you apply this to any customer interaction, whether it’s email, chat, or digital forms, it becomes quite unclear when the AI agent ends and where a human agent might begin. However, it becomes more clear when I ask it a particular question without giving it the details of my business:
In this instance, ChatGPT hallucinated the $25 to $100 pricing. A focus on transparency and accountability is paramount to avoid potentially disruptive customer interactions like this one. As the lines blur between humans and AI, it may make sense for businesses to notify customers when they are interacting with a machine or person on the other side. We’ll need to build customer trust before we can begin fostering collaborative relationships between human agents and AI agents.
Explainability of AI Hallucinations
Above we saw ChatGPT give us actual numbers for rental car prices. How did it get this information? In the Generative AI space, we call this “hallucinating”. When large language models are trained on billions of pieces of content, this includes snippets on rental car agreements and their prices. When they are deployed for individual businesses, many of them can be fine-tuned to learn the specific information, but they never forget the information from their initial training set.
As we introduce AI assistants into our customer experience flows, we will need to troubleshoot when they get the wrong answer. AI Explainability is an emerging industry to help us better understand AI conclusions and what we need to do to fix them.
Hallucinating also becomes especially prevalent when the incentives of AI are misaligned with the intended business goals. Like children trying to outwit their parents, an AI can Reward Hack its way to optimize the best possible outcome on the metrics they are targeting while missing the overall business objective.
Enterprises will need to diligently monitor and fine-tune these models to ensure they do not stray too far from their business objectives. We will begin seeing new job categories around prompt engineering expertise to craft AI with ideal responses and stay in its lane.
Corporate Readiness
Beyond the engineering and execution of these systems, teams will have to also consider the broader impacts of releasing Generative AI across their business. Many institutions account to regulatory agencies that ensure customer data and trust is being upheld appropriately.
Just like any other vendor, AI systems will need to be evaluated for specific security and privacy laws. It’ll be extra important to secure and validate that AI models are not being trained on personally identifiable information. We will need a careful balancing act of fine-tuning models generically for anonymized customer sets and then adding additional fine-tuning for specific customer evaluations.
While it may seem to be more patient than your average person, we forget AI models are built around the representation of human society, something we know is far from perfect. We need to ensure these models are continuously trained with fair DEI (diversity, equity, and inclusion) inputs to avoid building systems with inherent biases and prejudice.
Legislations are beginning to codify laws to prevent companies from executing AI models with bias in areas like race, gender, or ability. In Europe, the AI Act classifies the risk and outright prevents some models from being used in certain industries with plans to take it even further. While here in the US, states like Colorado have created laws to prevent insurance companies from using specific external datasets in their models.
While it wasn’t mentioned during the summit, I came to the conclusion we are missing the financial tools to help companies looking to readily adopt AI models. Despite the cost-savings of implementing AI, there may be a balancing expense by incurring additional added risk. I expect there to be a real opportunity for the business insurance and risk industry to build risk assessment tooling and financial vehicles for managing AI that hallucinates and AI that improperly discriminates.
Responsible AI
With the incoming surge of Generative AI, we should expect companies previously apprehensive of implementing AI to adopt the technology. The companies winning the long game will focus on building customer trust by augmenting existing solutions rather than harnessing AI as a full replacement. These winners will build strong feedback loops to keep the AI models in check and focus additional non-technical resourcing to both the legal and risk branches of their business.
Time will tell how Generative AI entering the enterprise will play out, but if Salesforce's latest development Einstein GPT is any indication, even the largest companies will begin to adopt it into practice. Let's just hope they do so responsibly.
Special thank you to Austin Gunter and Adam Penly for the edits and pushing me to deliver a concise message.
ChatGPT was not used to write this blog post... this time...